Welcome to Artificially Real, the only life sciences blog dedicated to AI solutions expanding Real World Data research.
Understand the biggest trends in AI impacting the Life Sciences industry. Understand the business values companies are recognizing through the use of LLMs and RWD.
Learn about changes companies have to make to achieve their business goals. Hear about the latest regulatory changes impacting your business operations.
Learn about industry best practices for designing, implementing, operating, and managing AI programs effectively.
Hear about critical regulatory, compliance, and privacy requirements as they change.
Learn how colleagues and peers how tackled similar challenges.
Learn about the innovative AI initiatives being undertaken by fellow Life Sciences firms.
Understand the business goals, processes, changes, and advancements undertaken to realize measurable value from the programs.
See the impacts realized by these inititives.
Read reviews of vendors that offer valuable components needed for effective RWD, AI, and analytics programs.
Learn about the capabilities, functionality, and focus of different vendors. Understand their developments and progress. We continually update our vendor listings to reflect the latest details.
Tags

BUILDING SMARTER WITH LLMS: A SERIES
New Blog Series Alert: “Building Smarter with LLMs”
Life sciences companies are sitting on goldmines of Real World Data—claims, EHRs, patient notes—but struggle to connect the dots. Enter LLMs.
In our new 5-part series, we break down how IT leaders can design and implement enterprise-grade LLM solutions to:
✅ Accelerate evidence generation
✅ Unlock new clinical + commercial insights
✅ Ensure compliance & data privacy
✅ Unify data silos and boost R&D ROI
Whether you’re evaluating use cases or scaling existing AI investments, this is your end-to-end blueprint.
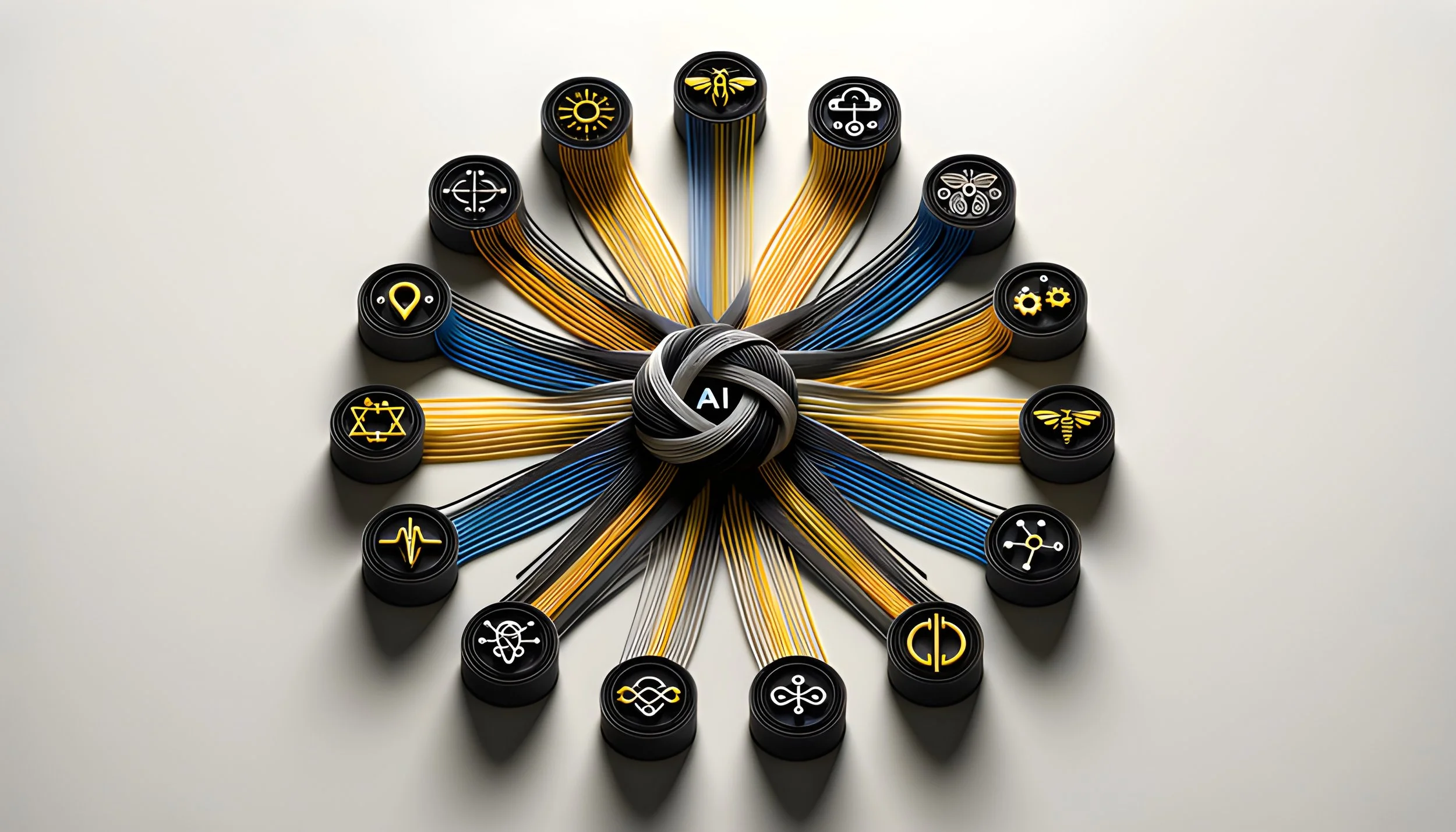
Interlnking Real World Data At Unprecedented Scale
Tthe fragmented and complex nature of RWD—spread across electronic health records (EHRs), claims data, clinical trials, and patient registries—poses significant challenges to effective analysis. Large Language Models (LLMs) are emerging as transformative tools for linking disparate RWD records, enabling life sciences companies to generate deeper insights and accelerate innovation
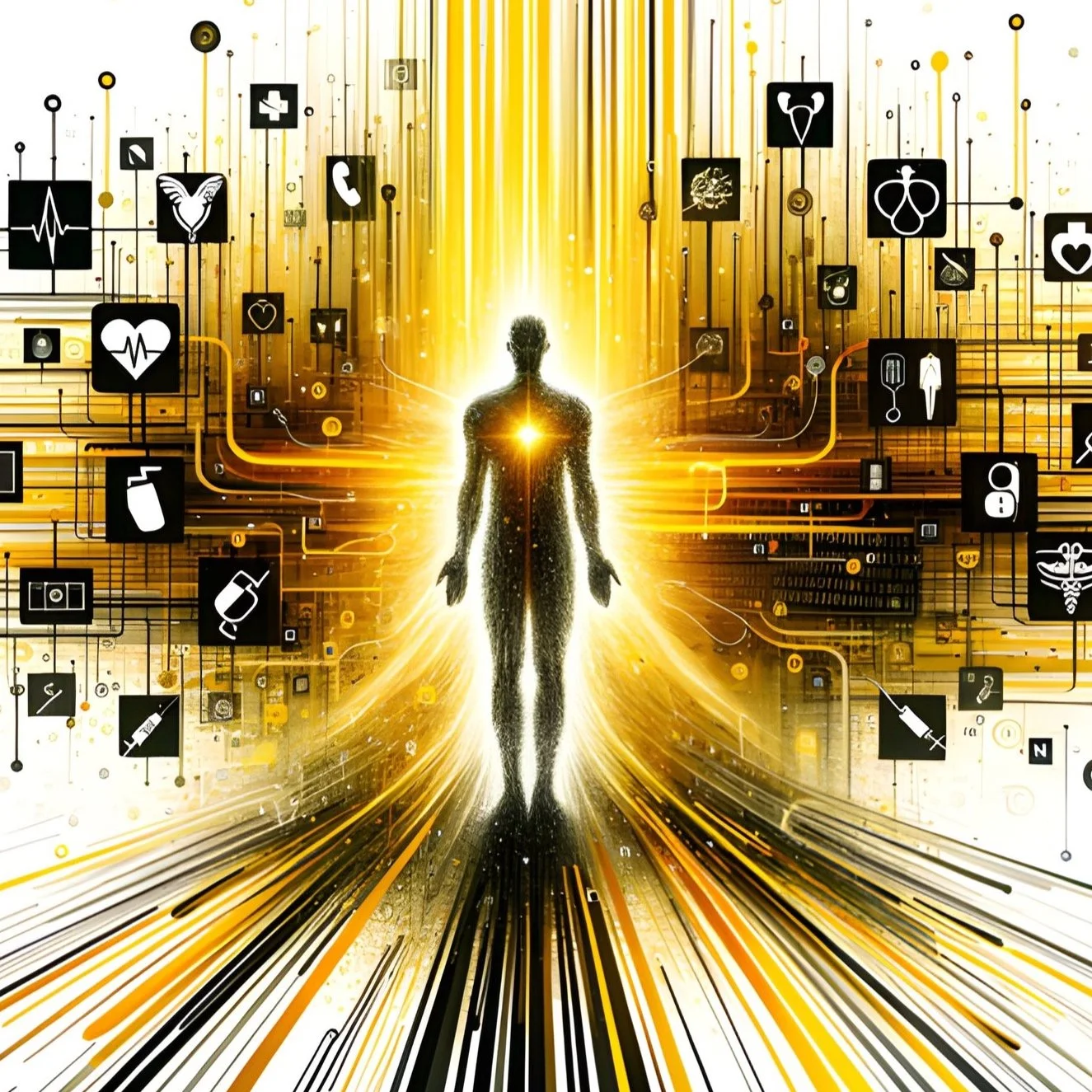
How AI is Expanding RWD for Clinical Trial Recruitment
Clinical Trials are the backbone of pharmaceutical innovation, driving the engine of development of new safe and efficacious drugs.
Artificial Intelligence (AI) is emerging as a game-changer in patient recruitment. Given the ability to process Real World Data (RWD) and other datasets rapidly, efficiently, and at scale, AI can deliver faster and more extensive data-driven insights. AI can streamline patient recruitment, identifying eligible patients, prioritizing those most suitable for specific trials, and even enhance recruitment efforts, ultimately making the process more efficient and cost-effective.

Innovative Ways AI is Changing Real-World Data Analysis
The explosion of real-world data (RWD) in life sciences, driven by new sources like wearable devices and social media, presents both opportunities and challenges.
Large language models (LLMs) offer a solution by enabling the analysis of vast amounts of structured and unstructured data, particularly in drug discovery, patient recruitment, adverse event detection, and analyzing social determinants of health.
Explore 5 innovative ways LLMs are transforming healthcare and drug development by accelerating innovation and improving patient outcomes.
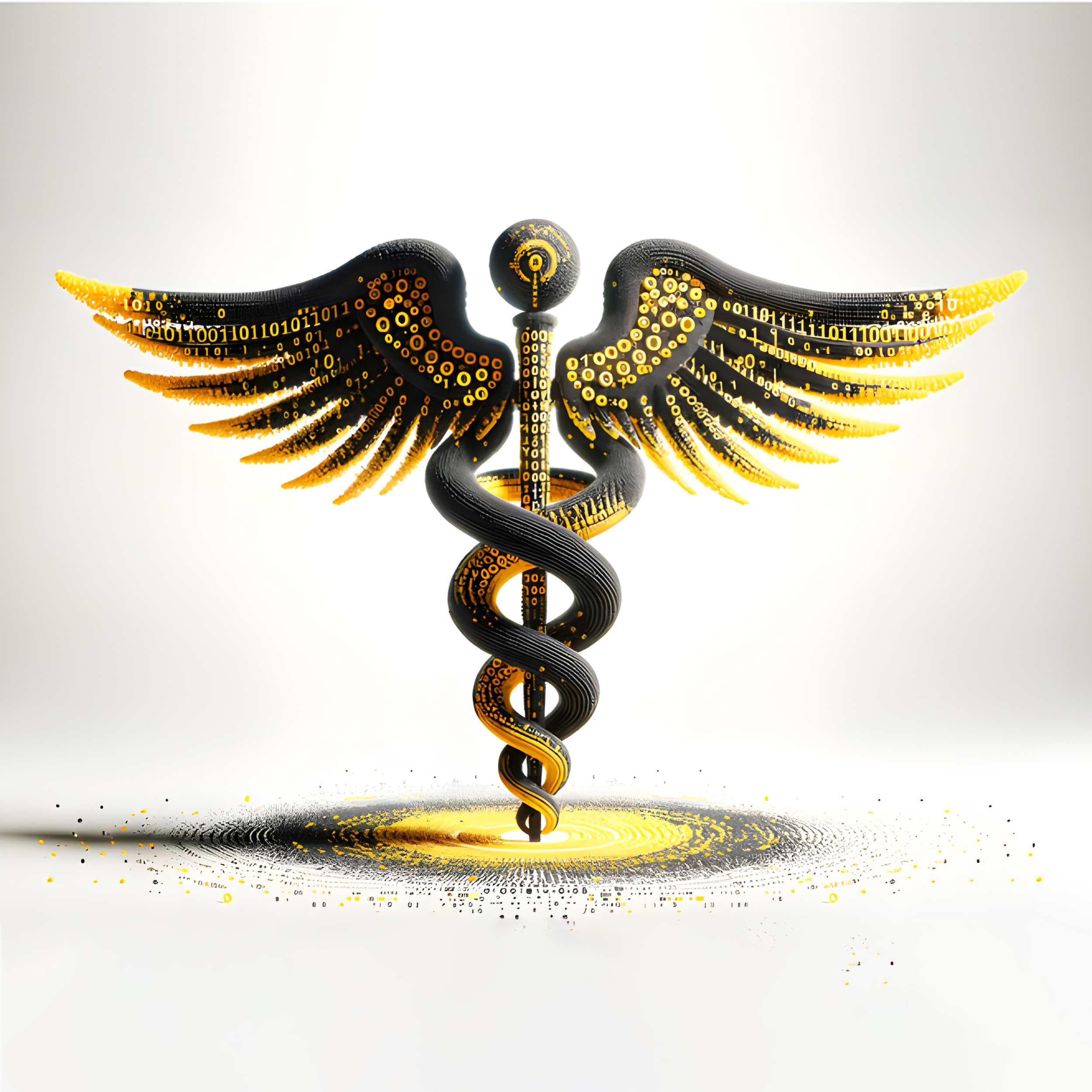
Enhancing Real-World Data Analysis: How LLMs Enable Advance Data Linkage
The life sciences industry relies heavily on real-world data (RWD) to drive research, improve clinical outcomes, and support regulatory decision-making. However, the fragmented and complex nature of RWD—spread across electronic health records (EHRs), claims data, clinical trials, and patient registries—poses significant challenges to effective analysis. Large Language Models (LLMs) are emerging as transformative tools for linking disparate RWD records, enabling life sciences companies to generate deeper insights and accelerate innovation.